Developments in the world of AI, specifically in the sub-field of machine learning, have led to quicker and more accurate insurance processes – but many in the industry are still in dark about how it works and how to apply it
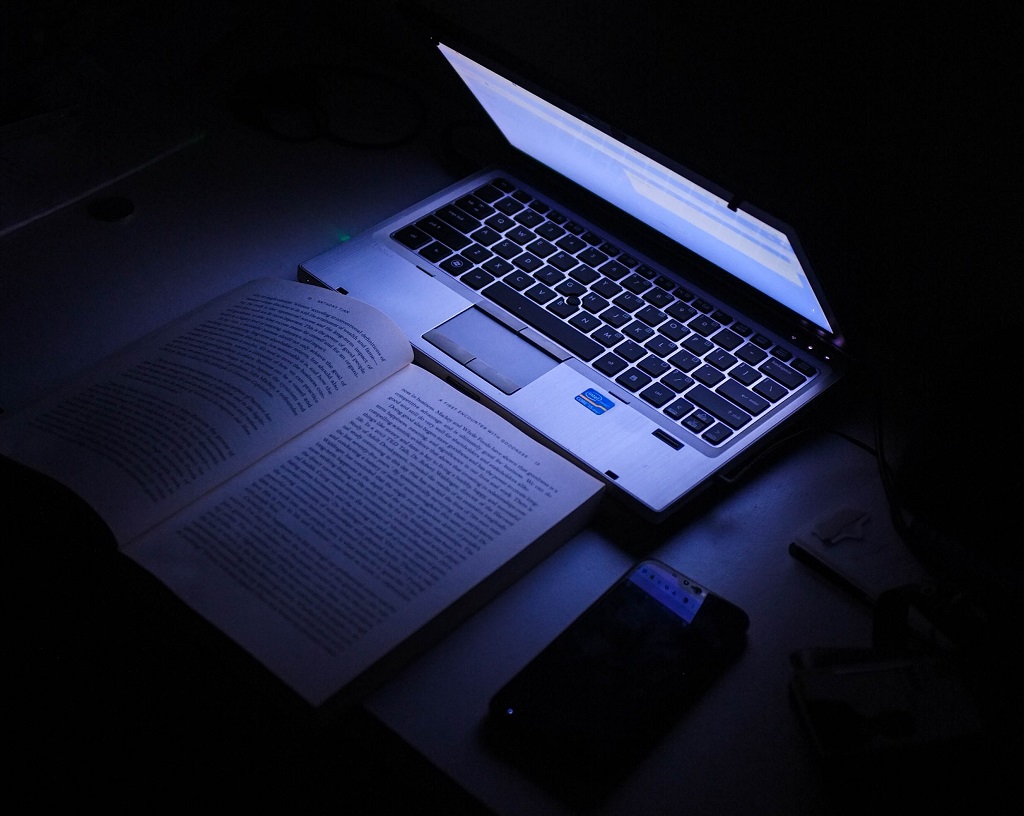
Artificial intelligence and machine learning are buzzwords constantly flying around in the insurance industry, as companies scramble to implement the latest technology and gain a competitive edge.
The rush to engage isn’t surprising when decision makers are seeing stats like AXA saving 18,000 man hours a year by allowing bots to take over repetitive tasks or watching satellite-based image recognition software that allows insurers to scan every property for risks, rather than the average 10% to 20% they were able to manually inspect before.
But many experts believe businesses misunderstand the fundamental value of using the tools, as well as the operational requirements and financial costs.
Speaking on a webcast hosted by global ratings agency AM Best, Patrick Sugent, vice president of analytics for Lexis Nexis Risk Solutions, says: “As machine learning techniques have gained a lot of popularity, and there’s a growing desire in the insurance marketplace use them.
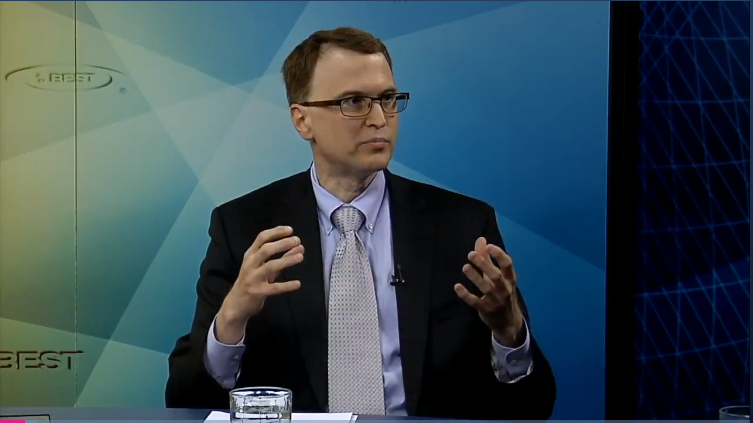
“We see some people who are less experienced with using them jump in and start running some of the software on their data without understanding all of the nuances that go into setting it up right and making sure the data is clean.
“It’s very easy to apply these types of tools and get an incorrect answer, and not even realise you’ve done that, unless you’ve got some experience with the pitfalls of using different types of techniques and how the data interacts with them.”
But for many businesses, technologies like AI and machine learning still need demystifying.
What is artificial intelligence and machine learning?
The field of artificial intelligence covers a wide spectrum of data science techniques, but in its simplest terms refers to the use of a computer system to perform tasks usually associated with the human brain.
Machine learning is a subset of AI that involves training a mathematical model to recognise patterns and execute decisions autonomously, rather than requiring an input command.
For Mr Sugent, part of the misunderstanding around AI and machine learning is driven by an unclear view of the subject matter and compounded by the proliferation of the terms within the industry.
He says: “Machines and people do different things and have different strengths. For example, people can be innovative and have a lot of domain expertise that allows them to be very intuitive.
“They can focus long-term and strategically, rather than having a short-term focus on local optimisations, and they understand intent better.
“We have people to do those jobs, and if we ever need more people, there are proven and popular methods of coming up with more.
“What we need machines to do is focus on the things they’re good at but people aren’t.”
What can machine learning technologies achieve in insurance?
One of the most important trends in insurance in recent times is the potential value of big data and the application of analytics.
Prior to advancements in AI, this meant analysing data points to create insurance ratings, which informed the pricing of policies – a process still widely used today.
Now, predictive analytics software can be used to reduce the time it takes to find correlations between data points in seconds, which Paulette Rice, senior director of analytics for Lexis Nexis Risk Solutions, sees as the real value of the technology.
“In the insurance industry itself, I really see machine learning in our day-to-day use in analytics for attribute development – so if I’m building a predictive model for underwriting risk for the telematics space, for example,” she says.
“Anything where you’re analysing data from sensors, whether it be on a vehicle, or now in the home, is going to be a wide use case for AI and machine learning.”
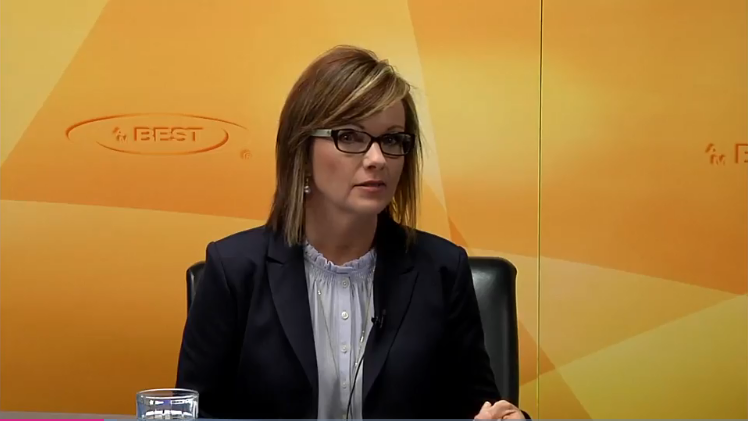
Mr Sugent believes the value of using AI and machine learning tools is intrinsically linked to the data that insurance firms wish to analyse, and they’d be wrong in thinking the technology can do all of the work for them.
“What’s really important to drive the success of all of these tools is the data that’s behind them, it’s definitely a garbage in, garbage out process.
“Getting the data together and organising it well, and having it ready for the types of tools to utilise is a critically important part of the process.
“It involves a lot of work and there may very well be data sets out there with a lot of value, but they might have been designed for a very different purpose than utilising machine learning tools.
“Some databases were designed for real-time reporting, and they’re very good at stating things exactly as they exist right now, but what’s important from a machine learning perspective is understanding not only how something looks right now, but how it got there.”
What do companies need to set up a machine learning process in insurance?
One of the key reasons AI and machine learning have exploded across industries is a reduction in the cost of using the technologies, fuelled both by the amount of open-source software available online and the proliferation of cloud storage as an alternative to expensive data warehouses.
Jeff Myers, US insurance giant State Farm’s analytics director, says: “The friction, or hurdle rate, has gone down so much that the return doesn’t have to be astronomical in order to apply it.
“It’s the same concept we saw when we had massive computers, and only the rich and famous or government bodies could afford them.
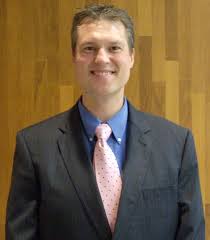
“Now, we all have the same processing power on our phones that we used to put someone on the moon.”
The real source of cost to a business, however, is its data science team, which Mr Myers believes is reasonable given the expected return from a company’s investment in one.
“A good data science and analytics team should be looking at a multiplier of about 13-times, and the amount for a small team is a couple of million, which individually would be prohibitive,” He adds.
“But for a company, it’s not more than other department.”
The importance of data scientists
For all three speakers, the business value unlocked by using AI and machine learning can only be realised with a high level of understanding provided by data scientists.
Ms Rice says: “Open-source is great, but you also need to understand what you’re using from that source, if you’re downloading a specific package written in programming languages like R or Python, you need to understand what they’re doing and some of the math behind them.
“Just because you can produce an answer, doesn’t mean it’s right or accurate, so knowing specifically what your programme is running, what you’re getting in your output, and having the ability to put that data somewhere is key.
“That requires some heavy data mining skills and an understanding beyond just skimming the surface of what you’re actually producing, and what that algorithm is doing for you.
“It’s a great thing to have as a tool, but don’t let it be your primary decision maker or a push button solution for you.”